
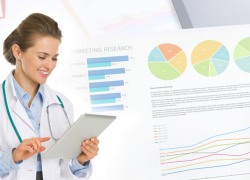
Human beings have always been fascinated with the ability to precisely anticipate the future, to shape it towards a more favourable outcome. With the emergence of massive amount of data, in addition to traditional business intelligence solutions, enterprises have now started evaluating predictive analytics. Predictive analytics is a sub practice of data science which processes past data to determine patterns, trends and estimate future outcomes.
This shift from business intelligence solutions to predictive analytics has opened a whole new market for established enterprises as well as many startup ISVs. According to a recent report from a research firm, MarketsandMarkets, Predictive analytics is projected to grow at a CAGR of 25.2% from 2013 to 2018 and reach up to $5.24 billion. There are various market areas for predictive analytics including financial intelligence, healthcare intelligence, data mining and management, and manufacturing etc.
This blogpost will cover some key advantages of predictive analytics in healthcare domain and dwell into the process of predictive modelling
Major Advantages of Predictive analytics in healthcare:
1- Cost reduction: Healthcare has been raking a huge expenditure, and has already crossed $3 trillion mark in 2014 alone. Predictive analytics can help in cost reduction by enabling a patient centric model, to improve care delivery and patient well-being. Hospitals can provide health prognosis to patients to define wellness programs. Also using patient historical data, hospitals can generate predictive analytics reports for insurance companies, to enable them to provide the most suitable plans for patients, which eventually results into cost reductions.
2- Improving chronic disease management
Based on the health records, Predictive analytics can detect if a patient is prone to any chronic diseases, and suggest proactive measures to improve the individual wellness accordingly.
3- Predicting outbreak of diseases
Based on the historic data and in correlation with the weather ailments, government bodies can predict if a particular strain of flu is about to break. This can help the state bodies in better planning – by broadcasting preventive measures to the public and stocking enough medicines.
Implementing predictive analytics
We need to define models to process raw healthcare data and exploit patterns that may be found in historical and transactional data. This will help in identifying risks and opportunities. Models capture relationships among many factors to allow assessment of risk or potential associated with a particular set of conditions, guiding decision making for candidate transactions. Hence predictive modelling is important in predictive analytics. Let us now look into the predictive modelling process in brief:
Above diagram illustrates schematic of the predictive modelling process. It starts with the raw healthcare data.
- Identify the problem which we want to address in Predictive analytics
- Explore raw healthcare data and identify the predictors, which are variables that can be measured for individuals or other entities, to predict required analytics
- Collect data according to the predictors identified and transform it into models by designing
algorithms - Validate and verify the models and tweak them for better accuracy
- Implement designed model to form predictive analytics
Most of the hospital EHR systems have Clinical decision support systems (CDSS), these systems can incorporate predictive analytics to support medical decisions made at the point of care. Also, using patient’s raw data like vital signs, problems, medications, allergies, assessments, care plan etc., we can implement predictive analysis in healthcare primarily to determine which patients are at risk. These predicted data can be provided to insurance companies, which further helps them recommend the best insurance plans for that particular area.
Let us now have a look on the common set of challenges faced by most organizations, as they go for implementation of predictive analytics:
- Massive Data: Some time it’s difficult to extract predictions from huge amounts of data. As data grows, we have to consider using massive scalable technologies, optimistic analytics algorithms and recommendation engine. Algorithms can be implemented using background processing jobs like MapReduce Jobs.
- Data Inconsistency: There might be issues in data collection, as data might be incomplete, heterogeneous, incorrect or inconsistent. Such analytics also need to address the problems of dealing large numbers of potential predictors and rarity of many of the events of interest. Data filtering and cleansing strategies should help overcome this challenge.
- Regulatory requirements: Healthcare applications face strict security and privacy compliance requirements. One should start implementing predictive analytics measures to comply with HIPAA/HiTech standards.
- Poor Documentation: Poor documentation results into human error or problems with EHR/EMR application. Ensuring data is clean and accurate before running analytics will naturally have a strong impact on the quality of the results. Reports and insights can only be as good as the quality of information that fuels them.
At Harbinger, we have worked on interesting cases in this domain. Predictive modelling in healthcare is at the forefront of improving quality of care, reducing costs, and improving population health. It has great potential to drive future models of care and is a key step towards personalized medicine.