
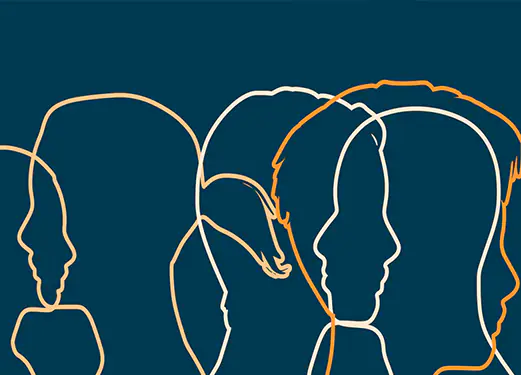
I read these lines somewhere. When technology integration is at its best, a student or an adult learner doesn’t stop to think that he or she is using a tech tool; it’s second nature. Technology must be like oxygen: ubiquitous, necessary, and invisible. Sounds a tad bit poetic but is totally relevant. This blog will look at how deep integrations play a pivotal role in ensuring technology becomes second nature. To experience the role of deep integrations in positively impacting student learning outcomes, let us navigate through the workflows within an AI-enabled skilling, reskilling, and upskilling ecosystem.
A research paper published by BenchPrep has revealed that 64% of learning providers surveyed have chosen learner performance as the number one business goal. Another research paper published by IBM highlights the importance of learning outcomes; it reveals that CEOs rank investment in the people as the number one way to accelerate performance. If we connect the dots, we can clearly see the plot; the learning outcomes will determine the executive buy-in. And it should be that way; it is indeed a win-win situation for all. In my last blog, we looked at the five different blocks of implementing an AI-enabled skilling ecosystem. A lot of these blocks constitute multiple software applications, platforms, which have been created by different vendors, and each one has its own technology stack and standards. To ensure the learning outcomes expected by the C- suite, we have to plumb these systems to ensure that they talk to each other, share their data across, provide actionable insights, and help impact the learning outcomes. Now we’ll peel the layers and see the role of integrations in the skilling ecosystem’s various workflows.
Workflow # 1: identifying the Competency Gaps
To achieve this, we need to run the performance data of the learner against the competency framework. In any typical scenario, the performance data would be getting captured in multiple systems, and each system would have its own standards and formats of capturing and storing data. Can we use this data from multiple sources and run it against the competency framework to identify the gaps? Ask this question to a data scientist, and you will get a big ‘NO’ as an answer. To make AI effective, we need data modelling, and to achieve that, we need standardization of data. So, we step back and build connectors to integrate these multiple systems and bring all the performance data to a central location in a standard format. And now we run that data against the competency framework to identify the gaps.
Workflow# 2: Recommending Learning Pathways
Now we move to the second workflow. To address the competency gaps, we need to recommend learning pathways. There is no dearth of good content, but we need to ensure that the learner doesn’t get buried under a stockpile of content sources and content delivery systems. Organizations have been focusing on ensuring they meet the learner at the point of the need. And to achieve this, they subconsciously ended up with multiple platforms and applications which deliver content. At times, the content formats also vary to a great extent. For example, apart from conventional eLearning, there could be VR labs, open educational resources (OER), PDFs, and more. Now, integration could tackle two key issues here:
- Improving usability and adoption. This will ensure that the learner is able to access most of the relevant content through a single sign-on.
- Transferring all learning outcomes to a central location and in a measurable format. This needs integration of the external content sources and the ancillary platforms and applications with the identified mothership or the central system.
Workflow #3: Providing Continuous Support in the Learning Journey
This one has undoubtedly been the most talked-about workflow. It started pre-pandemic as ‘making learning available in the flow of work,’ and as the pandemic evolved, the focus was on ensuring learner engagement. But I would say the underlying theme was the same, providing continuous support to the learning journey and impacting learning outcomes. Even though in workflow #2, we have been able to develop an integrated single sign-on platform that has curated learning pathways, but let us try and remember how many times in our everyday jobs have we gone to the LMS and searched for a specific learning quick fix. Now, what if we have an AI-enabled virtual coach which we can turn to for all our quick knowledge bites? The interface we chose to provide continuous support can vary from an AI-enabled smart bot to something else, but the key is to have two-way integration with the interface we chose. On one side of the integration strategy, we would have to provide access to the AI-enabled virtual to a dossier on the competency gaps identified for an individual and the recommended learning pathways. And the other side of integration strategy, we would have to make the smart bot available within the flow of work; it could be integrating with a collaboration tool like MS Teams, Slack, or with a CRM like Salesforce or HubSpot.
Using existing systems and integrating them in the right way can help identify competency gaps, recommend learning pathways based on the gaps, and provide continuous support to the learner at the point of need.
It would be interesting to know your thoughts on this topic. Are you using integrations in any way which is impacting learning outcomes? Are there any challenges that you are facing in integrating certain applications or platforms? Please feel free to write in the comments section below or drop a line at contact@harbingergroup.com.